Introduction
As a manufacturing leader, you understand the importance of predictive analytics in optimizing your operations. Two approaches that are gaining increasing attention are predictive maintenance and predictive quality. While both rely on machine learning and data analytics to drive insights and actionable intelligence, they have different objectives, benefits, and challenges. In this blog, we’ll explore the differences between predictive maintenance and predictive quality and explain how Vanti’s AI-powered no-code platform can empower subject matter experts to achieve predictive quality.
Predictive Maintenance: Asset Health, Performance, and Availability
The goal of predictive maintenance (PdM) is to foresee and avoid equipment failure using machine learning and data analytics. Using real-time data from sensors, IoT devices, and other sources, PdM keeps an eye on machinery and identifies any irregularities, patterns, or trends. Based on this information, predictive models can foresee impending breakdowns, empowering repair crews to take preventative measures.
Predictive maintenance’s main purpose is to increase the asset’s useful life, efficiency, and availability. Through the early detection of equipment failures, PdM serves to reduce downtime, increase asset utilization, and extend equipment lifespan. Moreover, PdM can help reduce maintenance expenses by facilitating more precise and economical preparation for maintenance.
There is no denying that anticipatory maintenance has many benefits. Seventy-six percent of manufacturing company heads surveyed found that PdM increased plant uptime, and 68% found that it decreased maintenance expenses. By identifying risks before they become actual dangers, PdM can also boost security.
However, there are challenges associated with PdM. It calls for a heavy financial outlay for infrastructure like monitors, Internet of Things (IoT) gadgets, and other data collectors. In addition, PdM requires the use of specialists with unique skill sets, such as data analysts and predictive modelers. Since PdM requires a transition from reactive to proactive maintenance, cultural barriers may also need to be surmounted.
Predictive Quality: Process Health, Product Health, Quality, and Performance
Predictive quality (PQ) is a data-driven approach to quality assurance that uses machine learning and data analytics to predict and prevent quality issues. PQ is a method of quality control that examines production-line metrics in real-time for outliers, trends, and patterns. Engineers and plant floor managers can take preventative action in the face of potential quality problems thanks to predictive models built on this data.
It’s hard to argue with the benefits of high predictive quality accuracy. PQ aids in lowering costs associated with errors, waste, and rework through early detection of quality issues and maintenance of constant high-quality output. PQ can help boost sales by reducing quality variation between production runs. Furthermore, the increase in quality due to PQ contributes to reduced production expenses.
Contrarily, PQ is not without its flaws. Like PdM, it requires a sizable financial outlay for infrastructure like monitors, IoT devices, and data storage. Experts in fields like data science and statistical modeling are required to achieve PQ. As PQ requires a transition from reactive to proactive quality control, a cultural change may also be required.
Comparing Predictive Maintenance and Predictive Quality
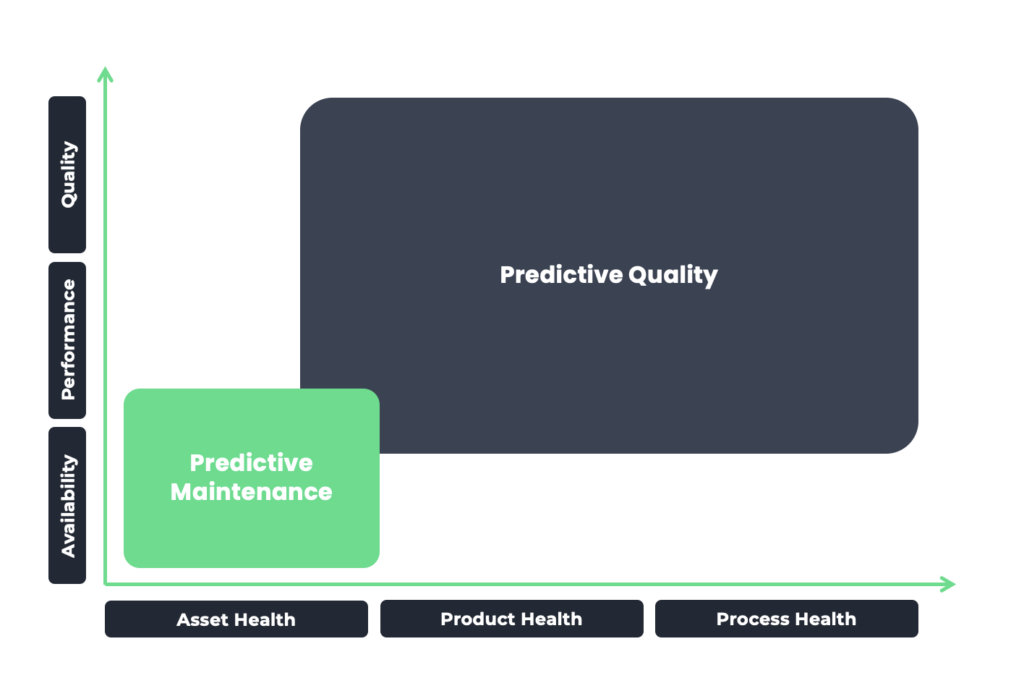
Key similarities and differences
While predictive maintenance and predictive quality have different primary objectives, they share many similarities in terms of the technology and data analysis methods they employ. Both approaches rely on machine learning algorithms and data analytics to identify potential issues before they occur, enabling proactive decision-making and preventive actions.
However, the focus of predictive maintenance is on equipment health and performance, while predictive quality focuses on product quality and consistency. Predictive maintenance uses data analysis to predict equipment failures and identify the root cause of problems, allowing for maintenance to be scheduled proactively. This results in reduced downtime and increased asset availability.
On the other hand, the main objectives of predictive quality are to focus on process health and product health, in turn enhancing quality and performance. Predictive quality analyzes production data to identify patterns, anomalies, and potential issues related to product quality. By proactively addressing potential quality issues before they occur, manufacturers can improve first-pass yield and ensure every batch is a “golden batch.”
There’s an interesting symbiotic relationship angle here: predictive maintenance can help prevent equipment failures that could negatively impact product quality, while predictive quality can identify potential quality issues before they impact equipment performance. By combining the insights gained from both approaches, manufacturing leaders can gain a comprehensive understanding of the overall health and performance of their operations.
Use cases and examples
There are many applications for predictive maintenance, including maximizing machinery uptime and preventing breakdowns by predicting and tracking the condition of vital systems. When it comes to jet engines, for instance, manufacturers like General Electric or Boeing may employ predictive maintenance to track how they’re doing so they can catch problems early and schedule repairs before they escalate.
On the other hand, predictive quality is used in a variety of industries, including the pharmaceutical and food and beverage industries, with the former using it to guarantee the constancy of product quality and the latter to reduce waste while maintaining or even increasing quality. Predictive quality can also be used by the automotive industry to boost product quality and cut down on revisions.
Impact on OEE (Overall Equipment Effectiveness)
Both predictive maintenance and predictive quality have a significant impact on OEE (Overall Equipment Effectiveness), a key performance indicator for manufacturing facilities. Predictive maintenance improves OEE by reducing downtime, increasing asset availability, and extending equipment lifespan. On the other hand, predictive quality improves OEE by reducing product defects, minimizing scrap wastage and line rework effort, and ensuring quality consistency across batches.
Vanti’s AI-Powered No-Code Platform for Predictive Quality
Vanti’s platform uses machine learning and data analytics to identify potential quality issues in real-time by analyzing production data. This improves first-pass yield, reduces waste, and ensures high-quality output. Vanti’s no-code solution empowers subject matter experts, like engineers and plant floor managers, to access and utilize the platform’s insights without requiring data science expertise or IT support, enabling quick deployment.
The platform offers real-time alerts to minimize defects and improve product quality, along with root cause analysis to take corrective actions and minimize waste. The analytics dashboards provide a comprehensive overview of production data, helping manufacturers spot patterns and make informed strategic decisions. With Vanti’s platform, manufacturers can achieve predictive quality and improve their OEE.
Industry Use Cases for Predictive Quality
Industries are constantly looking for ways to improve their manufacturing processes to increase efficiency, productivity, and quality. With the help of predictive quality solutions, manufacturers can achieve these goals by identifying quality issues early in the production process and making corrective actions before defects occur. Vanti’s AI-powered no-code platform is an excellent tool for subject matter experts to achieve predictive quality. Let’s take a closer look at some industry use cases for predictive quality using Vanti’s platform:
CPG and Food & Beverage Industry:
The CPG and food & beverage industries require strict quality control measures to ensure their products meet consumer expectations. With Vanti’s platform, manufacturers can use machine learning and data analytics to inspect products in real-time and identify discolored packaging, misaligned labels, or other defects. This helps manufacturers identify quality issues early on, reduce waste, and improve overall product quality. For instance, an oral hygiene product brand can use an AI system to detect defects in toothbrush production, such as missing bristles or misshapen heads. By leveraging real-time alerts and root cause analysis, engineers and plant floor managers can quickly identify root causes and make necessary changes to avoid production defects.
Semiconductor Industry:
In the semiconductor industry, manufacturers need to maintain high levels of precision and quality to produce reliable components. With Vanti’s platform, manufacturers can use machine learning and data analytics to predict possible problems before they happen. By analyzing data from thousands of sensors in real time, the platform can identify areas that could benefit from process optimization, such as adjusting temperature or pressure levels for better component performance. This helps manufacturers prevent assembly errors and improve overall product quality.
Automotive Industry:
The automotive industry is another industry that benefits from predictive quality solutions. Manufacturers can use Vanti’s platform to predict and stop problems before they happen, which helps find and fix quality problems early and reduce the chance of expensive recalls. The platform can monitor the production of various types of cars, including sports or SUV models. By looking at information from thousands of sensors in real time, the platform can identify quality issues early in the production process and make necessary changes to avoid production defects. This helps lower the risk of assembly mistakes and improves the overall quality of the product.
Conclusion
In conclusion, predictive maintenance and predictive quality are two critical technologies that have the power to revolutionize the manufacturing industry. While predictive maintenance aims to optimize asset health and ensure maximum uptime, predictive quality focuses on ensuring consistent, high-quality production, and minimizing defects and product recalls. Both technologies have similar underlying principles but have different use cases and impacts on OEE.
To achieve predictive quality, Vanti’s AI-powered no-code platform empowers subject matter experts to identify potential issues, predict quality problems, and take corrective action before they occur. The platform’s real-time alerts, root cause analysis, and analytics dashboards are powerful tools that help manufacturers streamline their production processes and improve their overall product quality. Vanti’s platform is applicable in several industries, including CPG, food and beverage, semiconductors, and automotive.
Predictive quality is a game-changer for the manufacturing industry, and Vanti’s AI-powered no-code platform is the key to unlocking its full potential. By embracing this technology, manufacturers can achieve tangible business outcomes, stay competitive in the market, and ensure consistent, high-quality production.